Efficient condition monitoring through vibrations
AIQ-Bo: Smart sensor autonomously detects anomalies
Experts from the Fraunhofer Cluster of Excellence Cognitive Internet Technologies CCIT have developed a smart sensor that measures vibrations in mechanical components and analyzes them on-site using artificial intelligence (AI). This makes it possible to identify anomalies autonomously and transmit this information to the cloud so appropriate corrective action can be taken.
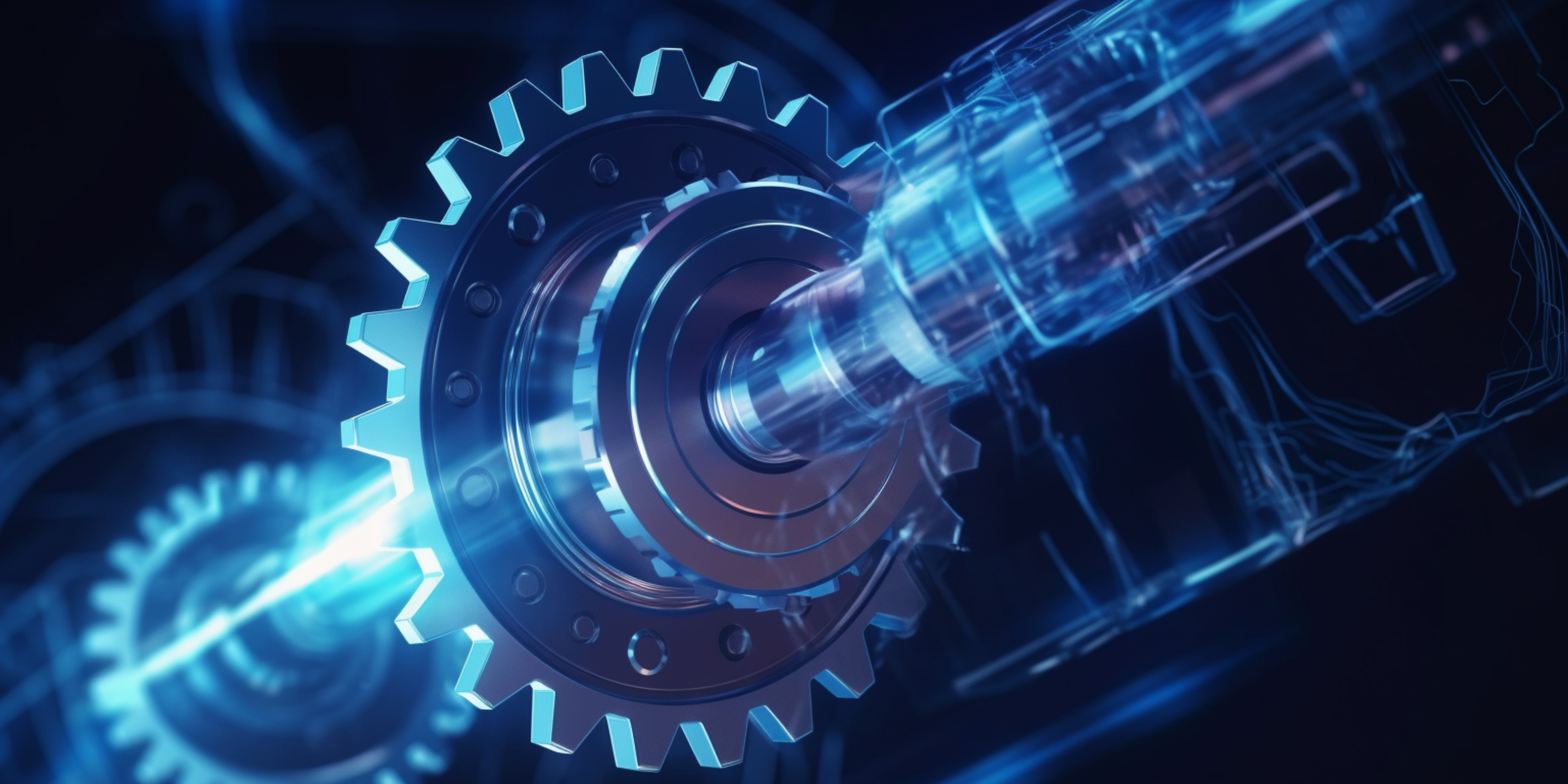
Detecting and fixing defective components, initiating maintenance, planning for replacements — and preventing costs and damage in the process. In their project “AIQ-Bo – AI enhanced Intelligent — Bolt,” researchers at the Fraunhofer CCIT have integrated a trainable AI model that identifies anomalies autonomously into a vibration sensor. The innovative part is that the AI is operated right at the sensor, so it detects discrepancies right at the site where the data generation takes place. This enables a forward-looking kind of condition monitoring — energy-efficient, decentralized, and space-saving.
Analysis directly in the edge device
Vibrations are both indications and, often, the cause of wear and damage affecting drive systems. In AIQ-Bo, an optimized AI model inside a three-axis acceleration sensor analyzes the vibration data logged. With this approach, the researchers at the Fraunhofer CCIT have made it possible to use AI in a microcontroller to analyze sensor data energy-efficiently and locally, right at the exact site.
The key advantage is that there is no need to transmit the vibration data to the cloud for analysis first. The AI runs on the device, and the information is processed using Fraunhofer’s embeddif.ai technology right where it is first generated: in the edge device. The trained AI model in the sensor’s integrated microcontroller autonomously detects whether the component is running in normal operation or may be reaching a critical state — all without sending data to the cloud. Status information is not relayed to the cloud wirelessly unless and until there is an issue. This dramatically reduces the volume of data that needs to be transmitted while also making the system extremely energy-efficient.
All this is made possible by an open source AI framework called AIfES®. The network can be used to operate and train artificial neural networks (ANNs) on almost any kind of hardware in IoT devices — like the simple microcontrollers used in this application.
Wireless AI retraining from the cloud
Computation-intensive tasks such as training the AI algorithm and calculating a new AI model are handled in the cloud. Model parameters can be updated and sent back to the sensor wirelessly to adjust the anomaly detection to factors such as changing environmental conditions.
The highly energy-efficient mioty® wireless technology is used to transmit the data. Energy harvesting supplies the sensors and wireless system with energy from their immediate surroundings, using things like temperature and sunlight to generate power. Small batteries can also be used as an alternative power source.
Energy efficiency and cost effectiveness with AIQ-Bo
“Fraunhofer CCIT develops technology solutions for the edge cloud continuum, which links decentralized edge computing and central cloud computing together seamlessly. Data processing is automatic and takes place where it makes the most sense in terms of efficiency and economic viability: either in real time at the edge, close to end devices and sensors, or in a scalable manner in centralized data centers. AIQ-Bo is a prime example of how this adds concrete value in condition monitoring,” explains Michael Fritz, Head of Office of the Fraunhofer CCIT.
Automated, AI-supported condition monitoring with AIQ-Bo eliminates the need for manual checks of the system by technicians, which would not be feasible during live operation of equipment such as offshore wind farms and would therefore bring hefty downtime costs.
The innovative technological solution is also highly adaptable: “Our goal was to design AIQ-Bo to be so flexible that it can be used with as little training as possible on different systems, including wind turbines, bridges, and overhead cranes,” says Dr. Peter Spies, the project manager responsible at the Fraunhofer Institute for Integrated Circuits IIS. Training in the cloud and the wireless transfer of data can also be translated to different application scenarios.
Project partners
The project run by the Fraunhofer Cluster of Excellence Cognitive Internet Technologies CCIT also involved the Fraunhofer Institute for Integrated Circuits IIS, which supplied its embeddif.ai technology and expertise in energy harvesting and efficient communication and made it possible to demonstrate the new system in a lab environment. The new solution also uses the AIfES® open source AI framework from the Fraunhofer Institute for Microelectronic Circuits and Systems IMS, which also worked on optimizing the AI models. Another partner, the Fraunhofer Institute for Wind Energy Systems IWES, was responsible for taking vibration measurements on various testing units and wind turbines. The Fraunhofer CCIT spokesperson role is performed by the Fraunhofer Institute for Applied and Integrated Security AISEC.
More informationen
- Learn more about embeddif.ai (iis.fraunhofer.de)
- Learn more about AIfES (Artifical Intelligence for Embedded Systems) (ims.fraunhofer.de)
- Learn more about mioty® – The Wireless IoT Technology (iis.fraunhofer.de)